Managing financial risks with machine learning algorithms
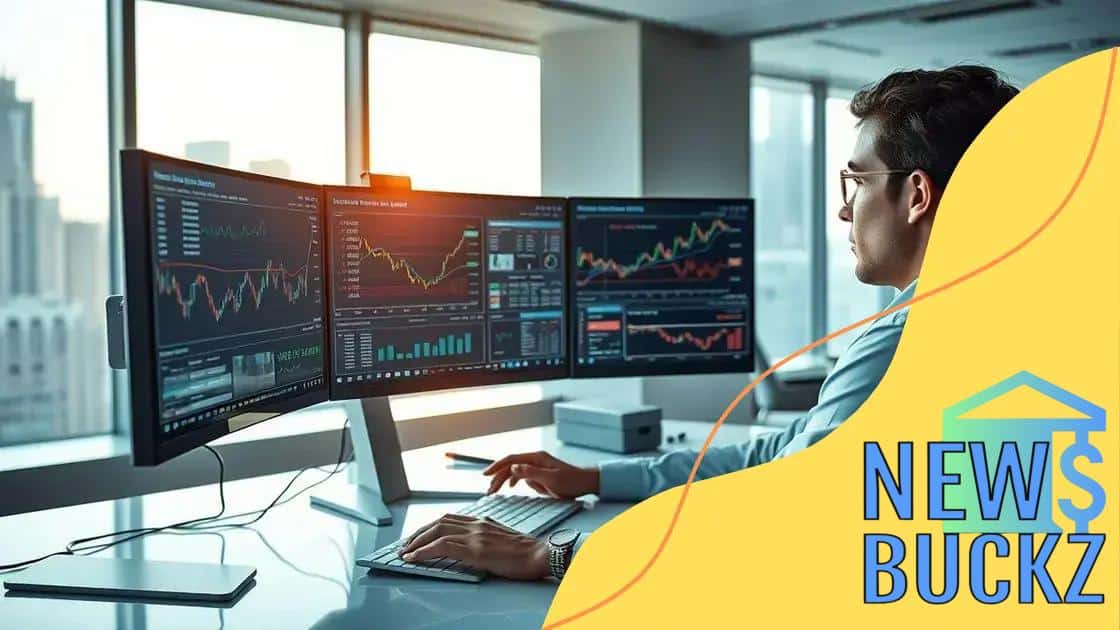
Managing financial risks with machine learning algorithms enhances predictive accuracy, automates risk assessment processes, and enables organizations to respond to market changes more effectively.
Managing financial risks with machine learning algorithms is becoming a game-changer in today’s unpredictable market landscape. Have you thought about how these technologies can transform your financial strategies?
Understanding machine learning in finance
Understanding how machine learning works in finance is essential for modern businesses. Financial institutions are gradually realizing the potential of these advanced algorithms to enhance their decision-making processes.
What is Machine Learning?
Machine learning refers to a branch of artificial intelligence that enables systems to learn from data and make decisions without being explicitly programmed. In finance, it allows analysts to forecast trends, assess risks, and make strategic recommendations.
Key Concepts of Machine Learning in Finance
- Data Analysis: Machine learning algorithms analyze vast amounts of data more efficiently than traditional methods.
- Predictive Analytics: These algorithms can predict market trends and consumer behaviors based on historical data.
- Risk Assessment: Machine learning aids in identifying and mitigating financial risks through sophisticated models.
- Fraud Detection: Improved detection of fraudulent activities is possible through pattern recognition capabilities of machine learning.
As we delve deeper into how machine learning is applied, we see that it helps automate trading processes. Algorithms can analyze market conditions and execute trades in milliseconds, outperforming traditional human traders. Additionally, financial institutions utilize machine learning for customer insights, tailoring services to meet individual needs based on transaction patterns.
Furthermore, machine learning improves portfolio management. By examining historical trends, it enables better allocation of assets, optimizing financial returns while managing risk. As these algorithms evolve, the finance industry adapts, leveraging their capabilities for improved efficiency.
In summary, a solid grasp of machine learning fundamentals is vital for anyone in finance. The ability to interpret data, predict outcomes, and manage risks can set businesses apart in a competitive landscape.
Key algorithms for financial risk management
Key algorithms for financial risk management play a crucial role in today’s fast-paced market. By leveraging the power of machine learning, these algorithms can help identify and mitigate various financial risks effectively.
Popular Algorithms in Financial Risk Management
Several algorithms are commonly utilized for assessing risk in financial contexts. These include:
- Decision Trees: These algorithms help in making decisions based on various input parameters, providing clear visualizations of risk assessments.
- Random Forest: This ensemble method combines multiple decision trees to improve prediction accuracy, making it ideal for complex financial datasets.
- Support Vector Machines (SVM): SVMs are effective in classifying data points into different categories, allowing for better risk classification.
- Neural Networks: These deep learning techniques model intricate patterns in data, identifying latent risks that might go unnoticed.
The use of these algorithms enhances the capability to manage risk proactively. For example, financial institutions often implement predictive analytics to foresee potential market downturns. This foresight enables organizations to adjust their strategies accordingly, thus safeguarding their investments.
In addition to individual algorithms, combining multiple approaches can yield even better results. A hybrid model, which integrates various algorithms, often provides a more comprehensive view of financial risk. This approach takes advantage of the strengths of each method, leading to more accurate predictions and analyses.
Financial risk management is no longer just about mitigating losses; it’s about creating effective strategies to capitalize on opportunities. By using key algorithms, firms can navigate uncertainties with greater confidence and make informed decisions.
Case studies of successful applications
Case studies of successful applications in financial risk management showcase how machine learning can drive impactful results. Numerous organizations have adopted these advanced algorithms to enhance their risk assessment processes.
Bank of America: Fraud Detection
One prominent example is the Bank of America, which implemented machine learning to improve its fraud detection systems. By analyzing transaction patterns in real-time, the bank has significantly reduced fraudulent activities, saving millions of dollars each year. This system learns continuously, evolving to identify new fraud patterns as they emerge.
American Express: Credit Risk Analysis
Another fascinating case is American Express. They utilize sophisticated algorithms to assess credit risk more effectively. By considering various customer data points, such as transaction history and spending behavior, they can accurately score potential clients. This method allows them to offer tailored products to customers while minimizing defaults.
- Real-time insights: Instant feedback helps in making quick decisions.
- Increased efficiency: Automating analysis reduces manual intervention.
- Improved accuracy: Enhanced predictive capabilities reduce risks.
Moreover, several hedge funds have adopted machine learning models to optimize their trading strategies. Using historical data, these funds can predict market movements and adjust their portfolios accordingly. By leveraging algorithms, they gain an edge over traditional methods, achieving higher returns with reduced risk.
In essence, these case studies demonstrate that implementing machine learning algorithms in financial risk management can lead to significant improvements in efficiency, accuracy, and profitability. Companies that embrace this technology are not only staying competitive but also paving the way for innovative solutions in the industry.
Challenges in implementing machine learning
Implementing machine learning in financial risk management presents several challenges. Understanding these challenges is essential for companies that want to leverage this technology effectively.
Data Quality and Availability
One major hurdle is the availability and quality of data. Machine learning algorithms depend heavily on large datasets to learn and make accurate predictions. If the data is incomplete, outdated, or biased, it can significantly affect the model’s performance. Companies must ensure they have access to high-quality data that is relevant to their specific needs.
Integration with Existing Systems
Integrating new machine learning systems with existing financial infrastructures can also be challenging. Many organizations have legacy systems that may not easily support advanced technologies. A seamless integration is vital to enhance workflow and ensure that the new tools can communicate effectively with existing processes.
- Employee Training: Staff must be trained to use these new systems effectively, which requires time and resources.
- Change Management: Resistance to change among employees can hinder the adoption of new technologies.
- Cost of Implementation: Financial investments are necessary for acquiring technology and training staff.
Furthermore, regulatory compliance also plays a role in the challenges faced during implementation. Financial institutions must navigate a complex landscape of regulations that govern the use of data and algorithms. This requires a continuous effort to keep up-to-date with regulations and ensure that all practices remain compliant.
As organizations embrace machine learning, it is essential to recognize and address these challenges proactively. By doing so, they can mitigate risks and fully realize the potential benefits of this powerful technology.
Future trends in financial risk management
Future trends in financial risk management are evolving rapidly. With the advent of new technologies, financial institutions are adapting their strategies to stay ahead of risks and market changes. Understanding these trends helps companies make informed decisions and enhance their risk management practices.
Increased Use of Artificial Intelligence
One significant trend is the increased use of artificial intelligence (AI) and machine learning. These technologies enable organizations to analyze vast amounts of data effectively. AI algorithms can identify trends and anomalies that humans may miss, leading to better risk predictions.
Real-time Risk Assessment
Another promising trend is the move towards real-time risk assessment. Companies are utilizing advanced analytics to monitor risks as they occur, allowing for quicker responses. This shift not only enhances risk management but also improves overall decision-making.
- Automation: Automating risk management processes reduces manual errors and saves time.
- Predictive Analytics: This approach uses historical data to forecast future risks, providing valuable insights for businesses.
- Regulatory Technology: Fintech solutions are emerging to help firms comply with regulations more efficiently.
Additionally, collaboration between firms is becoming more critical. Sharing data and insights can lead to a more comprehensive understanding of risks across the industry. This collaborative approach helps in developing industry-wide standards and practices that enhance overall financial stability.
The emphasis on sustainability is also shaping financial risk management strategies. As environmental, social, and governance (ESG) factors gain importance, companies will increasingly consider these elements in their risk assessments. This trend reflects a broader understanding that sustainability impacts financial performance and risk exposure.
In summary, the future of financial risk management will focus on leveraging technology, enhancing collaboration, and incorporating sustainability into risk strategies. These developments will help organizations navigate an ever-changing financial landscape.
FAQ – Frequently Asked Questions about Managing Financial Risks with Machine Learning
What are the main benefits of using machine learning in financial risk management?
Machine learning improves predictive accuracy, automates processes, and enhances data analysis, helping organizations manage risks more effectively.
How can data quality affect machine learning outcomes?
Data quality is crucial because incomplete or biased data can lead to inaccurate predictions, impacting the overall effectiveness of machine learning models.
What are common challenges in implementing machine learning?
Common challenges include data integration, employee training, technology cost, and ensuring compliance with regulations.
How is sustainability impacting financial risk management?
Sustainability is increasingly important as organizations consider environmental, social, and governance (ESG) factors in their risk assessments and strategies.