Advances in AI-powered predictive maintenance for industries
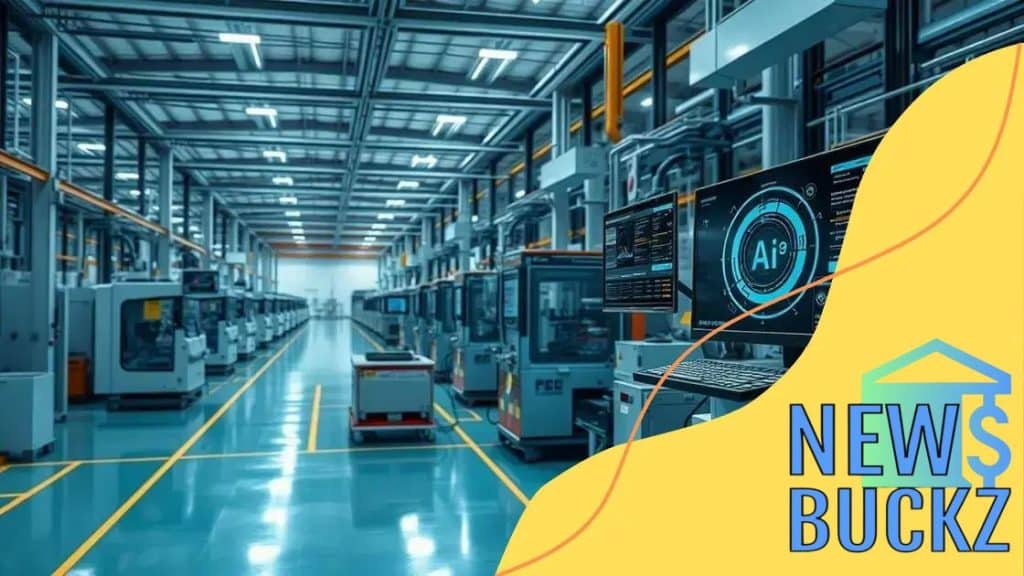
Anúncios
Advances in AI-powered predictive maintenance enable industries to anticipate equipment failures, reduce downtime, and optimize maintenance schedules, leading to significant cost savings and enhanced operational efficiency.
Advances in AI-powered predictive maintenance for industries are reshaping how businesses operate. Have you ever wondered how technology can predict failures before they happen? In this article, we’ll delve into the cutting-edge developments that are making this possible.
Anúncios
Understanding predictive maintenance
Understanding predictive maintenance is essential for staying ahead in today’s industrial landscape. This approach leverages advanced technology to anticipate equipment failures before they disrupt operations. Companies can save time and resources by adopting these techniques.
What is Predictive Maintenance?
Predictive maintenance refers to techniques designed to help determine the condition of in-service equipment to predict when maintenance should be performed. This helps avoid unexpected equipment failures and minimizes downtime.
How Does It Work?
This method utilizes data analytics and monitoring tools to assess the health of machinery and equipment. Here’s how it generally works:
Anúncios
- Data Collection: Sensors gather data from machinery.
- Data Analysis: Advanced algorithms analyze the data.
- Predictive Insights: The system predicts when maintenance should occur.
By predicting issues before they arise, industries can enhance productivity and reduce operational costs.
Benefits of Predictive Maintenance
Implementing predictive maintenance offers several advantages:
- Reduces downtime by minimizing unexpected failures.
- Extends the lifespan of equipment.
- Optimizes maintenance scheduling.
- Improves safety by addressing equipment issues proactively.
As industries grow more digitized, understanding predictive maintenance becomes paramount. Organizations that grasp this concept can better navigate challenges and implement efficient strategies that drive success.
Key benefits of AI integration
Integrating AI into predictive maintenance brings numerous advantages, helping industries improve efficiency and cut costs. This integration allows for smarter operations, enabling companies to perform maintenance before issues disrupt production.
Enhanced Decision-Making
AI systems analyze vast amounts of data to provide insights that humans may miss. Equipped with these insights, managers can make informed decisions quickly. This capability leads to better resource allocation and reduced downtime.
Predictive Insights
By using AI, organizations can predict equipment failures based on historical data and ongoing analysis. Some of the notable benefits include:
- Reduced Costs: Fewer unexpected breakdowns lead to lower maintenance costs.
- Increased Equipment Lifespan: Addressing issues early extends machinery life.
- Improved Safety: Fewer equipment failures mean a safer working environment.
As AI integration continues to evolve, its ability to learn and adapt becomes more refined. This dynamic approach empowers organizations to maintain a competitive edge.
Streamlined Operations
Another significant benefit is how AI helps streamline operations. By automating routine maintenance tasks, teams can focus on strategic initiatives. This shift not only boosts productivity but also enhances job satisfaction among workers.
Additionally, AI tools can optimize maintenance schedules, ensuring that tasks are performed at the most convenient times. This scheduling minimizes disruption to production and maximizes equipment availability.
Real-world applications in various industries
Real-world applications of predictive maintenance powered by AI have transformed many industries. By leveraging advanced technology, companies can maintain equipment more effectively and reduce operational costs.
Manufacturing Industry
In manufacturing, predictive maintenance plays a crucial role. Companies utilize sensors to monitor machinery. This technology helps predict failures before they happen, ensuring production lines stay operational.
- Data Collection: Sensors gather real-time information.
- Analysis: Advanced algorithms identify potential issues.
- Scheduled Maintenance: Businesses can plan maintenance during off-hours.
This proactive approach minimizes downtime and maximizes efficiency.
Transportation Sector
The transportation industry also benefits greatly. For example, airlines use predictive maintenance to keep aircraft in top condition. By analyzing data from various sensors, they can forecast when parts may need attention.
In addition, logistics companies implement predictive maintenance on their delivery trucks to reduce unexpected breakdowns. This helps keep shipments on time and maintains customer satisfaction.
Energy Sector
In the energy sector, companies use predictive maintenance to monitor power plants and wind turbines. This monitoring helps prevent outages and ensures a steady supply of energy.
Through advanced analytics, businesses can determine the right time to service equipment, reducing risks associated with equipment failure.
Healthcare Applications
Healthcare equipment also utilizes predictive maintenance. Hospitals monitor machines such as MRI and CT scanners. This monitoring ensures that every piece of equipment operates correctly, ultimately saving lives.
Healthcare providers can reduce costly repairs and avoid downtime by addressing issues proactively.
Challenges in implementing AI-driven solutions
Implementing AI-driven solutions in predictive maintenance can be beneficial, but it also comes with several challenges. Understanding these challenges is vital for successful adoption.
Data Quality Issues
One of the main hurdles is ensuring data quality. If the data fed into AI systems is incorrect or incomplete, it can lead to inaccurate predictions. Companies need robust data collection methods to ensure reliable input.
High Initial Costs
Setting up AI-driven solutions often requires significant investment. This includes purchasing software, hardware, and training employees. Many organizations may hesitate due to the upfront costs involved.
- Budget Constraints: Limited funds can hinder the implementation process.
- Training Needs: Employees may need extensive training to effectively use AI tools.
- Time Investment: Implementing these systems can take considerable time, delaying potential benefits.
It’s essential for companies to weigh these costs against the long-term gains of adopting AI technologies.
Integration with Existing Systems
Another challenge is integrating new AI solutions with existing systems. Companies often use legacy systems that may not easily adapt to new technology. Successful integration requires careful planning and possibly upgrading current infrastructure.
There can also be resistance from employees who may be uncomfortable with the changes in their workflow. Ensuring a smooth transition involves addressing these concerns and providing adequate support.
Regulatory Compliance
Finally, organizations must navigate regulatory requirements when implementing AI solutions. Compliance with data protection laws and industry standards is crucial.
Companies need to ensure that they collect and use data responsibly, which can be challenging in an ever-evolving regulatory landscape.
Future trends in predictive maintenance
The future of predictive maintenance is bright, with several emerging trends shape its evolution. As technology advances, industries will continue to evolve their maintenance strategies to enhance efficiency.
Increased Use of AI and Machine Learning
One significant trend is the increased reliance on AI and machine learning algorithms. These technologies will enable systems to not only predict failures but also learn from new data to improve predictions continuously.
Integration with IoT Devices
Another key trend is the integration of Internet of Things (IoT) devices. These devices will enhance data collection by providing real-time insights into equipment performance.
- Smart Sensors: Equipped with advanced sensors, machines can transmit data regularly.
- Remote Monitoring: Technicians can monitor equipment remotely, reducing the need for physical inspections.
- Data-Driven Decisions: IoT will facilitate faster and more informed decision-making.
This trend leads to more proactive maintenance approaches, ultimately lowering costs and minimizing downtime.
Enhanced Data Analytics
As data analytics tools improve, businesses will leverage more sophisticated techniques to analyze vast amounts of data. Enhanced analytics will provide deeper insights into equipment behavior and performance patterns.
Organizations will be able to not only look at historical data but also predict future trends using advanced modeling techniques. This capability will allow for better planning and quicker responses to potential issues.
Focus on Sustainability
The trend towards sustainability will also influence predictive maintenance practices. Companies are looking to reduce their carbon footprint and improve overall sustainability. Predictive maintenance helps by minimizing waste associated with unnecessary repairs and replacements.
By focusing on efficiency, organizations can avoid overusing resources, contributing to sustainable practices.
FAQ – Frequently Asked Questions about AI-Powered Predictive Maintenance
What is predictive maintenance?
Predictive maintenance is a technique that uses data analysis tools to predict when equipment maintenance should be performed, allowing for proactive repairs and reduced downtime.
How does AI improve predictive maintenance?
AI improves predictive maintenance by analyzing large volumes of data to identify patterns and predict equipment failures before they occur, ensuring timely maintenance.
What industries benefit from predictive maintenance?
Many industries benefit from predictive maintenance, including manufacturing, transportation, energy, and healthcare, as it enhances operational efficiency and reduces costs.
What challenges do companies face in implementing AI solutions?
Companies face several challenges, including ensuring data quality, high initial costs, integrating with existing systems, and navigating regulatory compliance.