How machine learning is enhancing credit scoring models
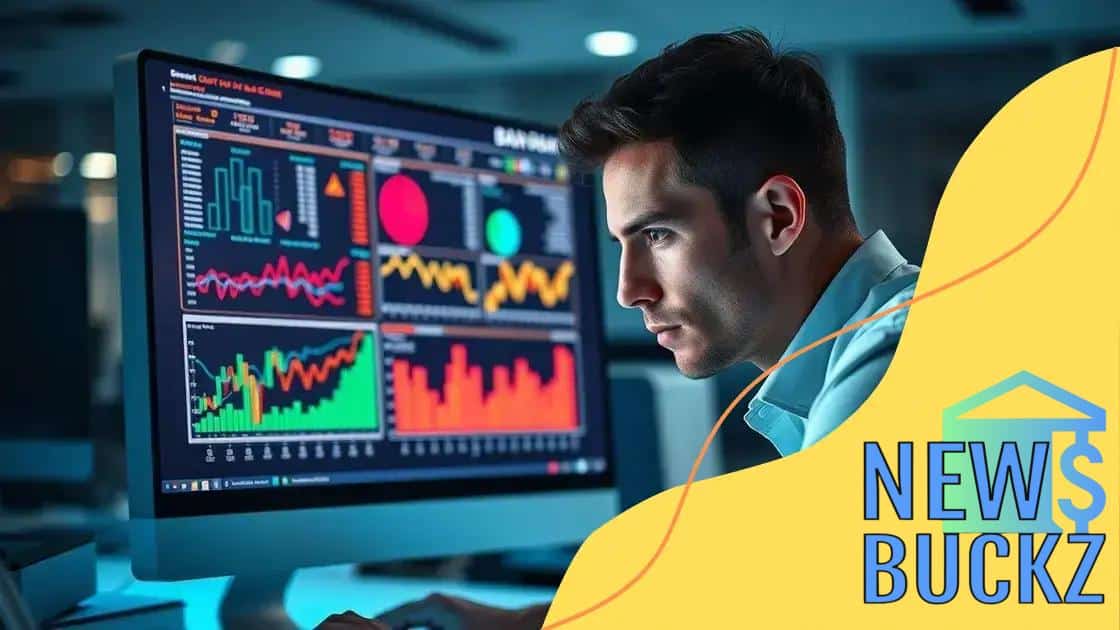
Machine learning is enhancing credit scoring models by increasing accuracy, enabling faster decision-making, promoting financial inclusion, and utilizing alternative data sources for more equitable assessments.
How machine learning is enhancing credit scoring models is transforming the financial landscape. Have you ever wondered how lenders decide who gets credit? Let’s dive into this topic.
Understanding machine learning in finance
Understanding how machine learning impacts finance is crucial in today’s technology-driven world. As financial institutions seek new ways to improve efficiency and accuracy, machine learning is proving to be a vital tool.
The Basics of Machine Learning
At its core, machine learning involves training algorithms on data so they can make predictions or decisions without human intervention. This capability has been embraced by various fields, especially finance.
Applications in Finance
- Risk assessment: Machine learning helps identify potential risks in lending and investment.
- Fraud detection: Advanced algorithms can spot unusual patterns that may indicate fraud.
- Customer insights: Machine learning analyzes customer behavior to offer tailored financial products.
The use of these applications not only streamlines processes but also enhances the accuracy of predictions. Institutions that integrate machine learning can adapt to market shifts rapidly, ensuring they remain competitive.
Furthermore, by leveraging large datasets, machine learning techniques can identify trends that traditional models might overlook. This provides a deeper understanding of both consumers and market dynamics.
Many companies are now using machine learning to refine their credit scoring models. They gather comprehensive data to create more equitable assessments of creditworthiness. These advancements contribute to a more inclusive finance ecosystem, enabling a broader range of individuals to access credit.
Machine learning is not without challenges, however. Financial institutions must ensure that their algorithms remain transparent and free from bias. Continuous monitoring and adjustment of these models are necessary to uphold fairness and accuracy.
In conclusion, understanding machine learning in finance opens up a world of possibilities. By embracing this technology, financial institutions can not only enhance their operations but also better serve their customers.
The evolution of credit scoring models
The evolution of credit scoring models has been remarkable, adapting to changes in technology and consumer behavior. Understanding this evolution is essential for grasping how today’s financial decisions are made.
Early Models
Initially, credit scoring relied heavily on manual assessments. Lenders would evaluate consumer creditworthiness by examining factors like income and employment history. This process was not only time-consuming but also prone to bias and inaccuracies.
Introduction of FICO Scores
In the 1980s, FICO scores were introduced. These scores consolidated data into a single number, making it easier for lenders to assess risk. The FICO model uses various factors, allowing for a more accurate prediction of creditworthiness. This shift marked a significant improvement in the lending process.
- Payment history: This accounts for 35% of the score, emphasizing the importance of timely payments.
- Credit utilization: It looks at the ratio of current debt to available credit.
- Length of credit history: A longer history generally contributes positively.
As technology progressed, lenders began incorporating more data sources into their algorithms. This expansion not only allowed for better risk assessment but also helped identify a broader range of customers, including those who might have been previously overlooked.
Furthermore, the rise of big data has transformed the landscape of credit scoring. Lenders today can analyze vast amounts of information in real-time. They can use alternative data such as payment patterns on utilities or rent to provide a more comprehensive view of a potential borrower’s financial behavior.
Current Trends
Today’s models continue to evolve, driven by advancements in machine learning and artificial intelligence. These technologies enable dynamic scoring models that adjust to changes in consumer behavior quickly and efficiently. As a result, credit decisions can be made in a matter of minutes rather than days.
This evolution has made the credit market more inclusive. Consumers with limited credit history or no traditional credit can still receive fair assessments based on their overall financial behavior.
The ongoing enhancement of credit scoring models is a testament to the adaptability of the financial sector. It reflects a commitment to utilizing technology to foster a fairer and more effective lending environment.
Key benefits of machine learning for credit scoring
The key benefits of machine learning for credit scoring are vast and impactful. By integrating advanced algorithms, financial institutions can enhance their credit assessment processes.
Improved Accuracy
One of the primary advantages is improved accuracy in predicting credit risk. Traditional scoring methods often rely on limited data, which can lead to incorrect conclusions. In contrast, machine learning analyzes large datasets, identifying patterns and correlations that human analysts might overlook.
Faster Decision Making
Another significant benefit is faster decision-making. With machine learning algorithms, credit scoring can happen almost instantly. This speed allows lenders to provide quick responses to loan applications, enhancing customer satisfaction.
- Real-time data analysis: Machine learning systems continually learn from new data, ensuring up-to-date assessments.
- Automated processes: Automation reduces human error and costs associated with manual reviews.
- Scalability: Machine learning models can easily adapt as the data grows, making them suitable for any scale of operation.
Furthermore, machine learning models reduce bias in credit scoring. Traditional methods can inadvertently favor certain demographics, leading to unfair treatment. Machine learning, on the other hand, applies objective criteria, enabling more equitable evaluations based on individual behavior.
The use of alternative data is also a noteworthy benefit. Machine learning allows lenders to incorporate non-traditional information, such as utility payments and rental history, into credit assessments. This inclusivity opens doors for many borrowers who might otherwise be excluded from the credit market.
Enhanced Risk Management
Risk management also sees dramatic improvements with machine learning. Algorithms can identify potential risks and fraud patterns much earlier than traditional methods. This proactive approach minimizes losses for lenders and creates a safer borrowing environment for consumers.
Ultimately, the key benefits of machine learning in credit scoring contribute to a more efficient, accurate, and inclusive financial system. As technology continues to advance, these models will likely become even more sophisticated.
Challenges in implementing machine learning
Implementing machine learning in credit scoring comes with several challenges that financial institutions must address. Understanding these challenges helps in navigating the complex landscape of modern lending.
Data Quality and Availability
One major hurdle is ensuring data quality. Machine learning algorithms rely on large volumes of accurate data to make precise predictions. If the data is incomplete or biased, it can lead to incorrect scoring outcomes, affecting lending decisions.
- Data collection: Gathering the right data from various sources can be time-consuming.
- Cleaning data: It’s essential to process and clean data to eliminate inconsistencies.
- Data privacy: With increasing regulations, protecting consumer data while using it for machine learning poses a significant challenge.
Another challenge involves the complexity of algorithms. Many machine learning models can be difficult to interpret. This lack of transparency makes it hard for lenders to explain decisions to customers, which is critical in maintaining trust.
Skill Gaps
There is also a notable shortage of skilled professionals who understand both finance and machine learning. Organizations need data scientists who can create and manage these models, and a lack of talent can slow down implementation.
Additionally, integrating machine learning into existing systems can be a daunting task. Legacy systems may not support the latest technology, requiring substantial updates. This can lead to increased costs and longer timelines for projects.
Furthermore, organizations must continuously monitor the performance of machine learning models. As market conditions and consumer behaviors change, models may need recalibration to remain effective. This ongoing adjustment can require significant resources, diverting attention from other important tasks.
Regulatory Compliance
Complying with regulatory standards adds another layer of complexity to machine learning deployment. Financial institutions must ensure that their models adhere to the legal and ethical standards set by governing bodies. Non-compliance can lead to hefty fines and reputational damage.
In summary, while the implementation of machine learning in credit scoring holds great potential, it is not without its challenges. Organizations must navigate data issues, algorithm complexities, skill shortages, integration hurdles, and regulatory requirements to capitalize on its benefits.
Future trends in credit scoring technology
The future trends in credit scoring technology promise exciting advancements that can reshape the lending landscape. Understanding these trends helps stakeholders adapt to evolving market conditions.
Integration of Artificial Intelligence
One major trend is the increased integration of artificial intelligence (AI) in credit scoring. AI enhances the predictive power of credit models by analyzing vast datasets that traditional methods simply can’t process. This means lenders can make more informed decisions based on a comprehensive view of borrower behavior.
- Personalized assessments: AI allows for customized credit evaluations based on individual circumstances.
- Dynamic scoring: Real-time data analysis enables lenders to adjust scores as consumer behavior changes.
- Fraud detection: AI algorithms can identify potential fraud patterns more effectively than standard methods.
Moreover, the adoption of blockchain technology is gaining traction in the credit industry. Blockchain can provide a secure and transparent way of verifying transactions and identities, reducing the risk of fraud. This technology can help create a decentralized credit scoring system where all parties have access to accurate and immutable data.
Emphasis on Financial Inclusion
Future trends are also highlighting the importance of financial inclusion. With many individuals lacking traditional credit histories, lenders are using non-traditional data points to assess creditworthiness. This shift broadens access to credit for underserved populations, ensuring that more people can enter the financial system.
Increasingly, financial institutions are leveraging alternative data such as rental payments, utility bills, and even social media activity to make lending decisions. This practice not only enhances the accuracy of credit assessments but also fosters greater financial accessibility.
Enhanced Consumer Engagement
Another trend is the focus on enhancing consumer engagement through technology. Mobile applications and online platforms are being developed to help consumers monitor their credit scores and receive personalized advice. This increased transparency empowers consumers to manage their finances better and improve their credit profiles.
Stakeholders in the financial sector must remain aware of how these emerging trends will affect their strategies and operations. By embracing innovation in credit scoring technology, lenders can improve their risk assessments and serve a broader customer base.
In conclusion, the evolution of credit scoring technology presents both exciting opportunities and significant challenges. As machine learning and AI continue to shape the landscape, they pave the way for more personalized, accurate, and inclusive lending processes. However, addressing inherent challenges is crucial for ensuring fairness and transparency in credit assessments. Stakeholders in the financial sector must stay informed about these developments to adapt and thrive in a changing environment.
FAQ – Frequently Asked Questions about Machine Learning in Credit Scoring
What is machine learning in credit scoring?
Machine learning in credit scoring refers to the use of algorithms to analyze large datasets and improve the accuracy of credit assessments.
How does machine learning improve credit scoring accuracy?
It improves accuracy by analyzing diverse data points and identifying patterns that traditional scoring methods may miss.
What challenges are associated with implementing machine learning?
Key challenges include ensuring data quality, regulatory compliance, and the need for skilled professionals to manage AI systems.
How can machine learning promote financial inclusion?
By using non-traditional data sources, machine learning can assess the creditworthiness of individuals who lack traditional credit histories.